

Mass spectrometry based peptidomics has become an attractive approach for developing peptide-based vaccines and immunotherapies. This field of research has also led to many discoveries of non-canonical peptide expression and has changed our understanding of the genomic regions that are read and translated. Assigning the correct amino acid sequence to an MS/MS spectrum is critical in peptidomics, since the positions and identity of each residue can influence peptide-protein interactions and define whether it is a good candidate for downstream analysis. With gaining popularity of peptidomic and immunopeptidomic analyses, it is essential to have a software tool that efficiently processes this type of data in an accurate and intuitive manner.
In the mass spectrometry analysis of peptidomics data, PEAKS Software has become the preferred data analysis solution and, as part of PEAKS Studio 11 and PEAKS Online 11, we have a workflow dedicated for this named DeepNovo Peptidome.
Key Features
- Integration of de novo sequencing, database search, and homology search in one workflow for both DDA and DIA data (optional LFQ and QC available)
- FDR control for de novo peptides
- Deep learning model trained with large HLA peptide dataset for retention time, fragmentation ion, and ion mobility predictions
- Three different categories of PTMs to predict the retention time and MS2 spectra of modified peptides more accurately
- Improved DDA Peptidome with additional Non-Canonical database searches.
- Integration of Proteomics and Genomics via a Gene tab.
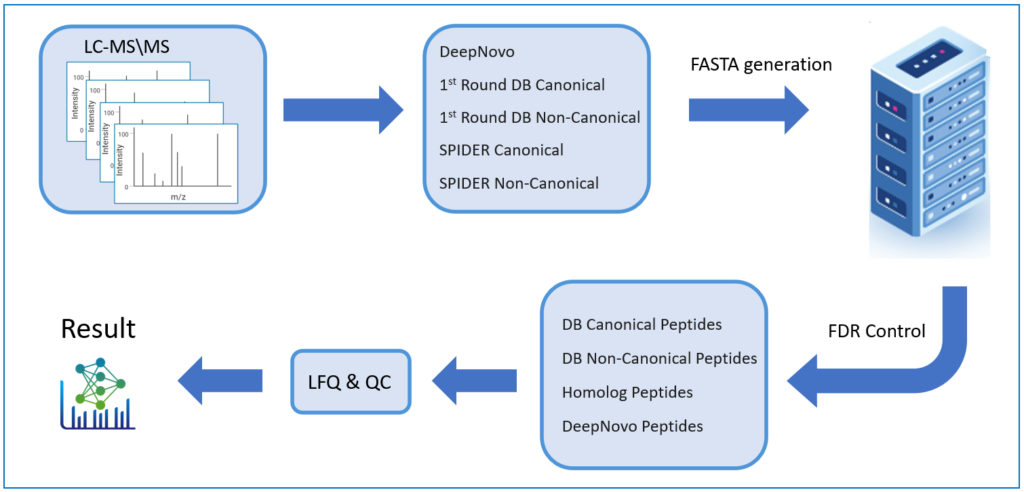
Previously, false discovery rate estimation was not an option for de novo sequenced peptides, therefore the user would choose an average local confidence score threshold to filter out poor quality. By appending de novo sequences to a protein sequence database and performing a second-round database search, FDR is estimated for de novo peptides to increase the accuracy of identification.
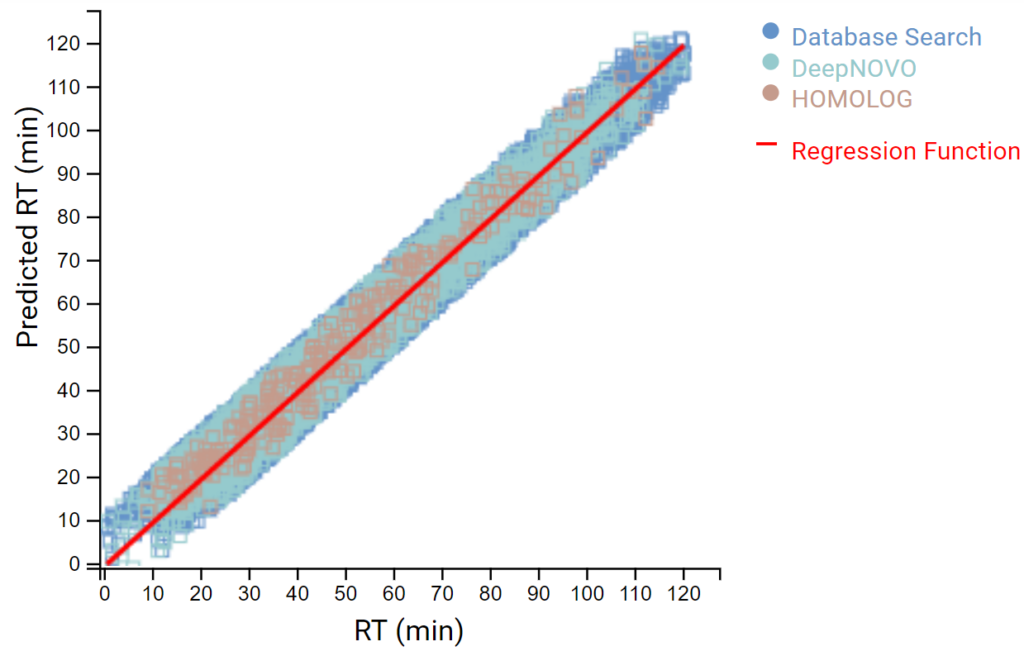
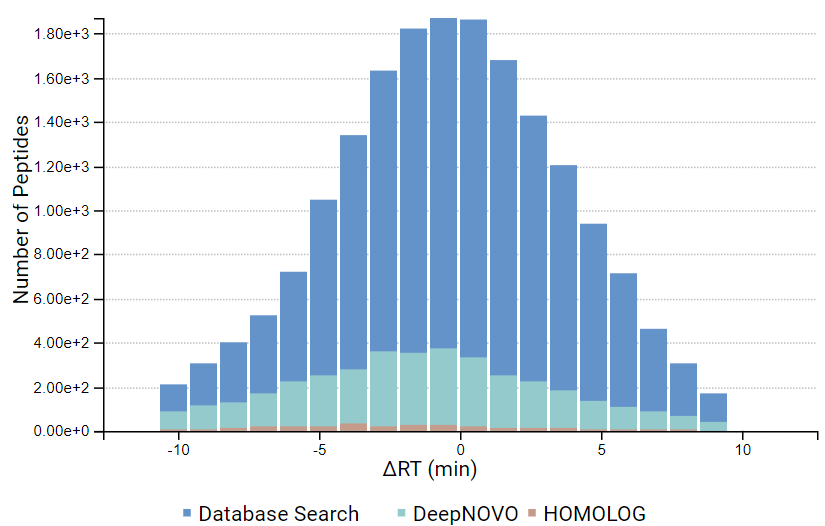
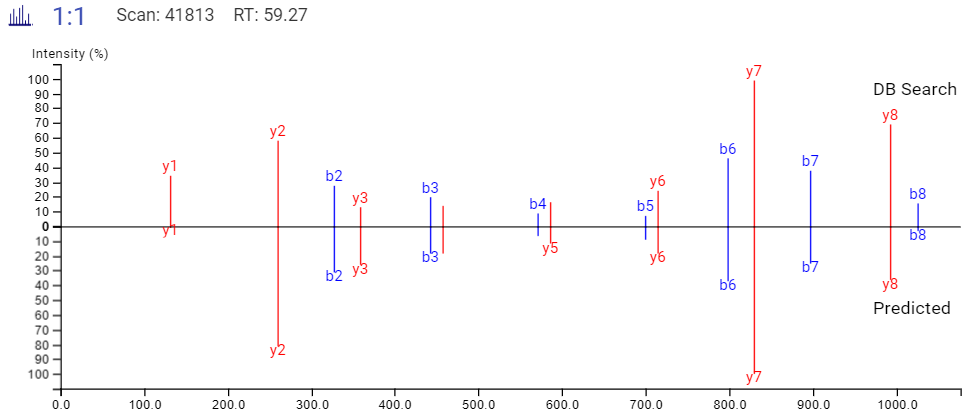
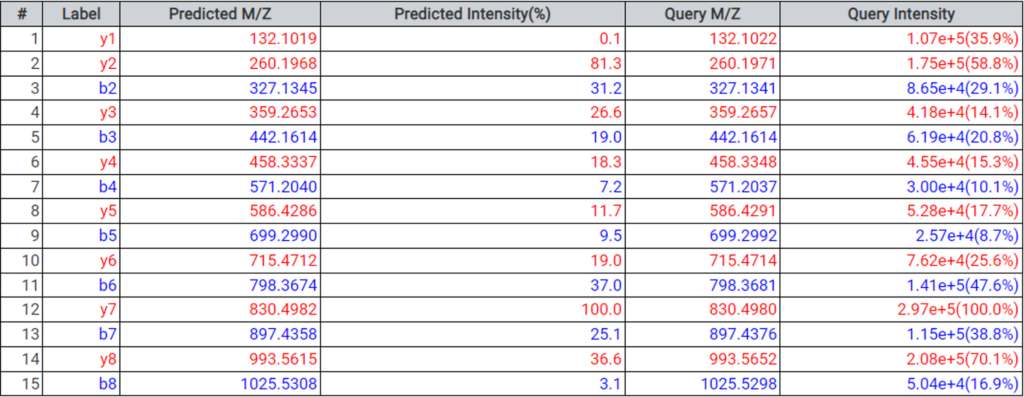
DeepNovo Peptidome provides a finalised list of peptides that can be sorted based on the way they were identified: database search, homology search (peptides with mutations), or de novo sequencing alone. The summary page of the results allows the user to evaluate how well the retention times of peptides from different classifications fit to the retention time predictions. By selecting any particular peptide in the result list, the m/z and intensity values for fragmentation ions in each spectrum can be compared to the predicted values. For ion mobility data, collisional cross section (CCS) values are also predicted for each peptide.
A new result filter, called confident amino acid percentage (CAA%), was added in DeepNovo Peptidome to allow the user to assess the percentage of amino acids in a peptide spectrum that are supported by fragmentation ions with intensity values above a specified threshold. This provides increased confidence in peptide sequence validation. The final peptide list can be directly exported and used for downstream analysis, such as binding affinity and immunogenicity predictions.
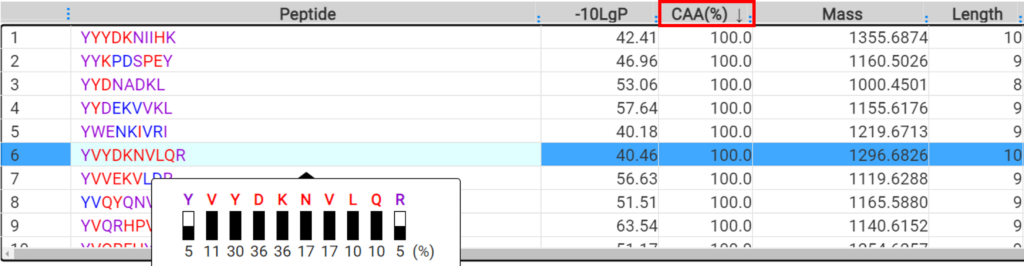
DeepNovo Peptidome PTM analysis
PEAKS DeepNovo peptidome considers three different categories of PTMs to predict the retention time and MS2 spectra of modified peptides more accurately. These categories include standard PTMs, isotopic labels, and artificial modifications (i.e., TMT tags). Isotopically labelled peptides are often spiked into immunopeptidomics samples prior to LC-MS/MS analysis to validate the identification of immunopeptides. However, amino acid isotopes do not affect retention time of peptides as do most biologically relevant PTMs or artificial tags. Therefore, the deep learning model in DeepNovo Peptidome workflow is trained to predict the retention time and MS2 spectra of peptides that fall into these three different categories to improve peptide scoring and identification.
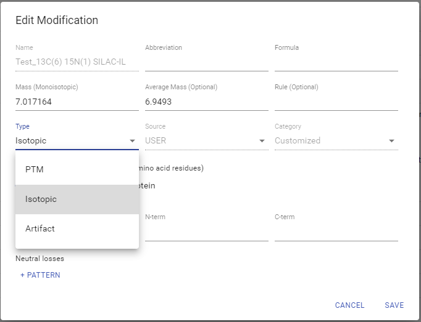
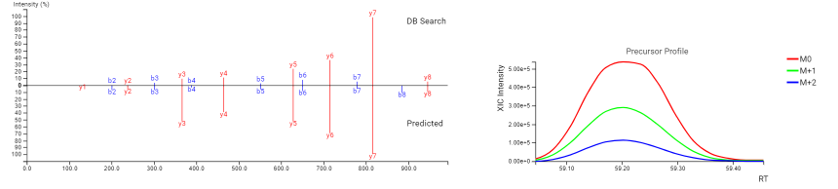
DeepNovo Peptidome DDA workflow expands the boundaries of Traditional Proteomics
Peptidome DDA workflow is designed to bridge the gap between proteomics and genomics through the integration of a comprehensive gene table.
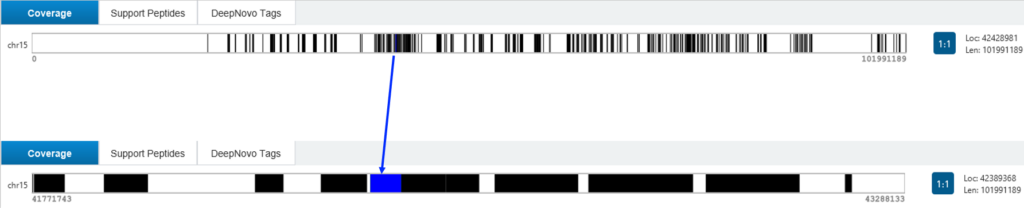
This feature facilitates a seamless connection between peptide identification and corresponding genomic data. The workflow supports non-canonical reference sequences, allowing for the identification of variant and modified sequences that are often missed by standard canonical reference databases.
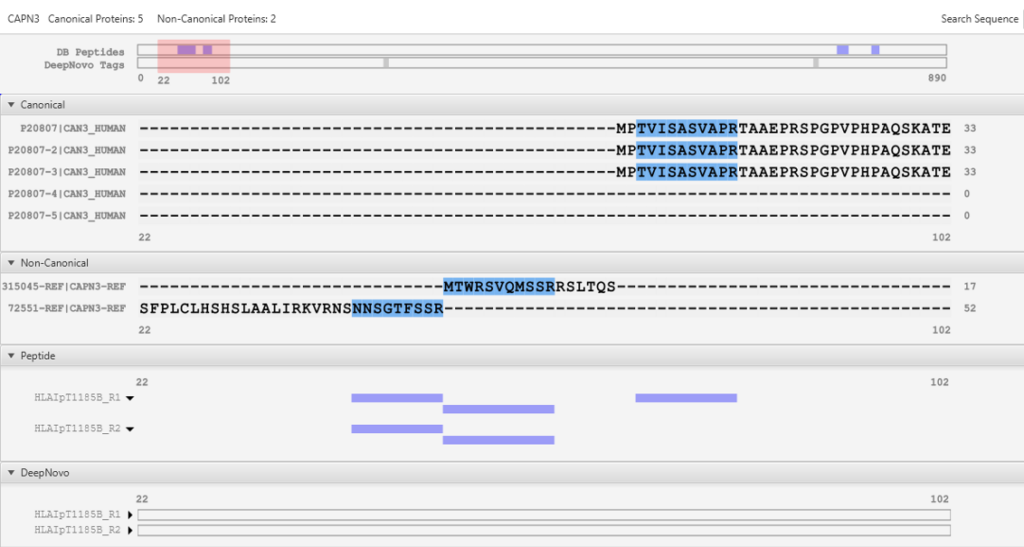
For quantitative analysis, the workflow includes robust label-free quantification capabilities, complemented by an automated QC tool to ensure data quality and reliability.
DeepNovo Peptidome for DIA Data
The new PEAKS 12 features a novel DIA peptidome workflow for immunopeptidomics that integrates library-free database search and de novo sequencing by stream-lined DIA data analysis [3]. Peptides with high confidence were collected and re-scored based on the prediction of peptide physic-chemical properties (including the fragment ion pattern, retention time, and ion mobility) and finally were reported with a false discovery rate. Furthermore, the positional confidence scores are associated with each peptide to evaluate the accuracy of each amino acid. The workflow presents a computational pipeline to aid identification of peptides generated by noncanonical translation of mRNA or by genome variants with LC-MS/MS DIA data.

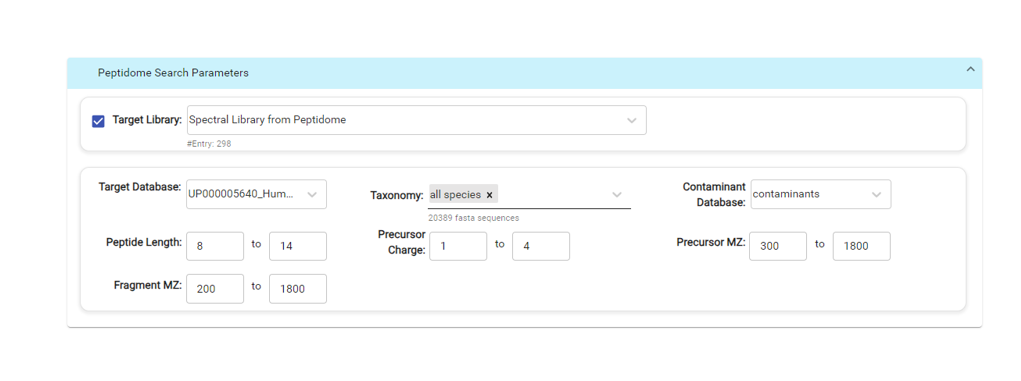
References & Resources
References
- Tran NH, Qiao R, Xin L, Chen X, Liu C, Zhang X, Shan B, Ghodsi A, Li M, Deep learning enables de novo peptide sequencing from data-independent-acquisition mass spectrometry, Nat. Methods, 16, 63–66 (2019). https://doi.org/10.1038/s41592-018-0260-3
- Tran NH, Zhang X, Xin L, Shan B, Li M. De novo peptide sequencing by deep learning. Proc. Natl. Acad. Sci. U.S.A. 114, 8247-8252 (2017). https://doi.org/10.1073/pnas.1705691114
- Xin, L. Qiao R, Chen X, Tran NH, Pan S, Robinoviz S, Bian H, He X, Morse B, Shan B, Li M. A streamlined platform for analyzing tera-scale DDA and DIA mass spectrometry data enables highly sensitive immunopeptidomics. Nat. Commun. 13, 3108 (2022). https://doi.org/10.1038/s41467-022-30867-7